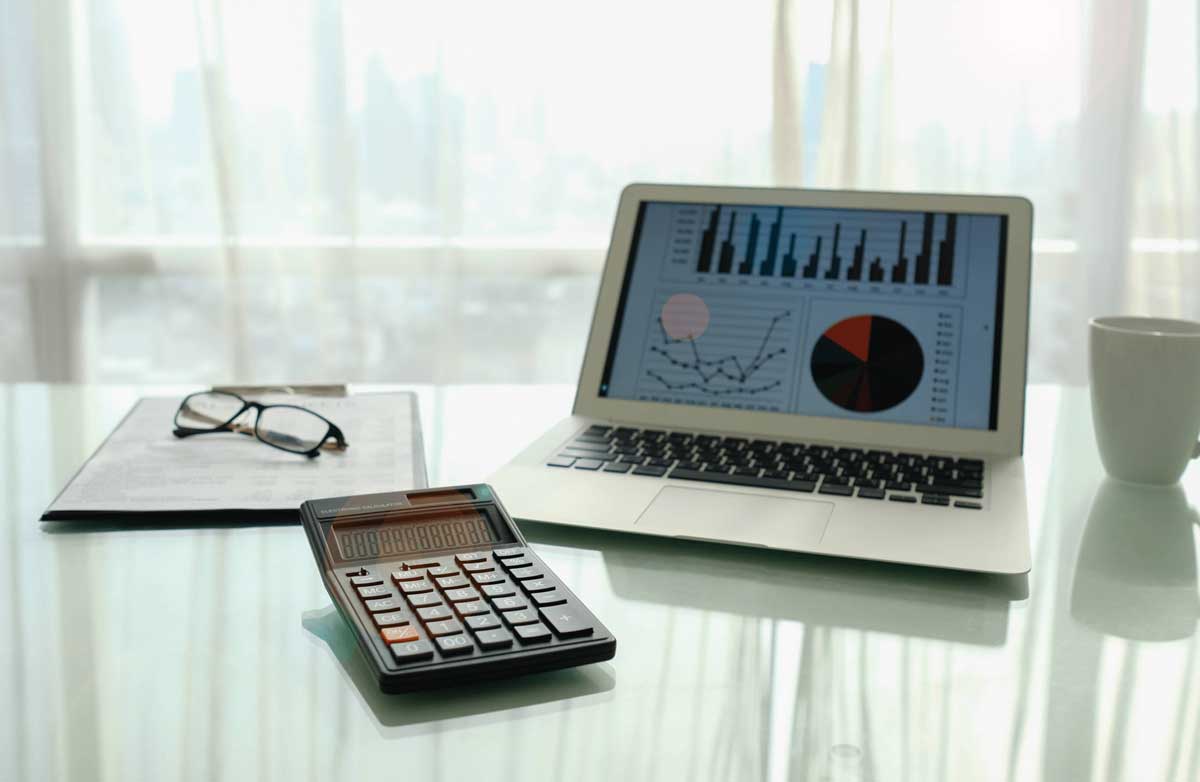
Data analytics and reporting can be beneficial for a business in various ways and at different stages of the business lifecycle. Some key instances when these tools are particularly helpful include:
Identifying trends and patterns: Data analytics can help businesses uncover hidden patterns, correlations, and trends in their data, which can be used to make better-informed decisions and improve operations.
Enhancing decision-making: By providing insights based on historical data, analytics can support data-driven decision-making, allowing businesses to make better and more informed decisions about their operations and strategies.
Improving efficiency and productivity: Analytics can help identify bottlenecks, inefficiencies, and areas where resources are underutilized, enabling businesses to optimize their processes and reduce costs.
Customer segmentation and targeting: By analyzing customer data, businesses can better understand their target audience and create more targeted marketing campaigns, which can improve customer acquisition and retention rates.
Predictive analytics: Data analytics can be used to forecast future trends, customer behavior, and sales, enabling businesses to anticipate challenges and opportunities.
Performance monitoring: Regular reporting and analysis of key performance indicators (KPIs) can help businesses track their progress toward goals and identify areas that need improvement.
Competitor analysis: By monitoring and analyzing competitors' data, businesses can identify gaps in the market, understand their competitive position, and make more informed strategic decisions.
Risk management: Data analytics can help businesses identify and mitigate risks, such as potential fraud or compliance issues, by monitoring and analyzing data for unusual patterns or anomalies.
Enhancing customer experience: By analyzing customer feedback and behavior, businesses can identify areas for improvement and take actions to enhance customer satisfaction.
Innovation and growth: Data analytics can help businesses identify new market opportunities, create innovative products or services, and explore potential growth strategies.
In summary, data analytics and reporting can be beneficial for a business at any stage, as they help drive informed decision-making, optimize operations, improve customer relations, and ultimately increase profitability.
What are the advantages and disadvantages of "In house" vs "Contracting a service"?
"In-house" and "contracting a service" each have their advantages and disadvantages when it comes to data analytics and reporting. Here's a comparison to help you decide which approach may be more suitable for your business:
In-house Data Analytics and Reporting:
Advantages:
Control: You have complete control over your data, analytics processes, and reporting, ensuring that your analysis aligns with your business objectives and priorities.
Customization: You can tailor the analytics and reporting to your specific needs and requirements, including proprietary tools or methodologies.
Faster communication: In-house teams can communicate and collaborate more easily, leading to faster decision-making and problem-solving.
Data security: Keeping data in-house can reduce the risk of data breaches and maintain better control over data access and privacy.
Skill development: Building an in-house team can help develop internal expertise and foster a data-driven culture within your organization.
Disadvantages:
Costs: Hiring, training, and retaining skilled data analysts and experts can be expensive, particularly if you require a diverse skill set.
Limited scalability: Expanding your in-house team to handle increasing data volumes or new projects can be time-consuming and resource-intensive.
Technology investment: You will need to invest in the necessary hardware, software, and infrastructure to support your in-house analytics and reporting processes.
Contracting a Service for Data Analytics and Reporting:
Advantages:
Cost-effectiveness: Outsourcing can be more cost-effective, as you only pay for the services you need and avoid the expenses associated with hiring, training, and maintaining an in-house team.
Expertise: Outsourced providers often have a broad range of skills and expertise, ensuring that your business has access to the latest techniques and best practices in data analytics and reporting.
Scalability: Contracting a service allows you to easily scale your analytics efforts up or down based on your needs and budget.
Time savings: Outsourcing allows your internal team to focus on core business activities while the service provider handles the data analytics and reporting tasks.
Access to advanced tools: Service providers typically have access to state-of-the-art analytics tools and software, which may be cost-prohibitive for some businesses to invest in.
Disadvantages:
Less control: Outsourcing can lead to a loss of control over your data analytics processes, and it might not always align with your business goals or priorities.
Data security risks: Sharing sensitive data with an external provider increases the risk of data breaches and privacy concerns.
Communication challenges: Working with an external provider may involve slower communication and decision-making, particularly if they are located in a different time zone or have multiple clients to manage.
Dependency: Relying on an external provider can lead to a dependency on their expertise and resources, making it challenging to transition back to an in-house approach if needed.
Ultimately, the decision to build an in-house team or contract a service for data analytics and reporting depends on factors such as your business goals, budget, data security needs, and internal resources. It's essential to carefully assess your organization's specific requirements and weigh the advantages and disadvantages of each approach before making a decision.
Once a detailed analysis is completed. What are the preferred methods for implementing changes reflected in the "Data analytics and reporting" performed? List 5 detailed methods often used.
After completing a detailed analysis using data analytics and reporting, it's crucial to effectively implement the insights gained to drive business improvements. Here are five methods often used for implementing changes based on data-driven insights:
Develop a data-driven action plan: Based on the insights gained from the analysis, create a comprehensive action plan that outlines the specific steps, goals, and timelines for implementing the necessary changes. This plan should include assigning responsibilities to team members, setting measurable targets, and establishing a clear roadmap for execution.
Prioritize changes based on impact and feasibility: Identify which changes can deliver the most significant impact on business performance and prioritize them based on their feasibility, required resources, and potential ROI. This can help you focus your efforts on high-value initiatives and avoid spreading resources too thin across multiple projects.
Communicate findings and involve stakeholders: Share the results of the data analysis with relevant stakeholders, such as team members, managers, and executives. Clearly explain the rationale behind the proposed changes and how they align with the organization's goals. Engage stakeholders in the decision-making process and solicit their feedback to ensure buy-in and support for the implementation process.
Integrate changes into existing processes and systems: To ensure a smooth implementation, integrate the proposed changes into your organization's existing processes, systems, and workflows. This may involve updating policies, procedures, or tools, or providing additional training to employees. Establish mechanisms for monitoring progress, tracking key performance indicators (KPIs), and measuring the impact of the implemented changes.
Continuous improvement and iteration: Data-driven insights should not be treated as a one-time exercise. Instead, adopt a continuous improvement mindset, regularly reassessing the implemented changes and their outcomes. Use ongoing data analytics and reporting to track progress, identify areas for further optimization, and make necessary adjustments. This iterative approach helps to refine and improve your strategies over time, ensuring that your organization remains agile and responsive to shifting conditions.
By adopting these methods, businesses can successfully implement changes based on data analytics and reporting, leading to improved decision-making, enhanced operational efficiency, and increased competitiveness.
Here are five well-documented case studies that demonstrate the successful implementation of data analytics and reporting to drive business improvements:
Netflix:
Netflix leverages data analytics to understand user preferences, tailor content recommendations, and make decisions about producing original content. By analyzing user behavior, ratings, and viewing history, Netflix can create a highly personalized experience for subscribers, which has contributed to its success as a streaming platform.
Case Study Reference: https://hbr.org/2014/10/how-netflix-reinvented-hr
UPS:
United Parcel Service (UPS) uses advanced data analytics to optimize its delivery routes and reduce fuel consumption. UPS's On-Road Integrated Optimization and Navigation (ORION) system analyzes package data, vehicle telematics, and route information to find the most efficient routes for drivers. This has resulted in significant cost savings and reduced environmental impact.
Case Study Reference: https://www.ups.com/us/en/services/knowledge-center/article.page?kid=aa3c4b6c
Coca-Cola:
Coca-Cola uses data analytics for market segmentation and targeting. They analyze consumer preferences and behavior to develop targeted marketing campaigns and create innovative products tailored to specific customer segments. One example is the launch of Cherry Coke in China, which was informed by data-driven insights on local taste preferences.
Case Study Reference: https://www.bernardmarr.com/default.asp?contentID=966
American Express:
American Express utilizes advanced data analytics to detect fraudulent transactions, identify customer preferences, and predict customer churn. Their predictive models analyze historical transaction data and customer behavior patterns to identify anomalies and proactively address potential issues, resulting in improved customer satisfaction and reduced financial risk.
Airbnb:
Airbnb uses data analytics to enhance customer experience and drive business growth. They employ data analysis to optimize pricing, personalize search results, and identify trends in user behavior. Additionally, they use natural language processing (NLP) and machine learning algorithms to analyze customer reviews and identify areas for improvement.
Case Study Reference: https://www.kdnuggets.com/2016/06/airbnb-data-science-machine-learning.html
These case studies demonstrate the power of data analytics and reporting in driving business improvements across various industries. By effectively implementing data-driven insights, these companies have achieved notable success in areas such as customer experience, operational efficiency, and market innovation.